In statistical analysis, the t-test is a powerful tool used to determine if there is a significant difference between the means of two groups. SPSS offers a user-friendly interface for performing t-tests, making it accessible to researchers and analysts across various fields.
Purpose of T-Test in SPSS
The primary purpose of conducting a t-test in SPSS is to assess whether the means of two groups are statistically different from each other. This analysis is commonly employed in research to compare the averages of variables across different conditions, treatments, or populations.
Types of T-Tests in SPSS
SPSS provides options for performing several types of t-tests, depending on the research question and the nature of the data. The most common types include:
- Independent Samples T-Test: Used when comparing the means of two independent groups.
- Paired Samples T-Test: Applied when comparing the means of two related groups or conditions (e.g., before and after measurements).
- One-Sample T-Test: Used to determine if the mean of a single sample differs significantly from a known or hypothesized population mean.
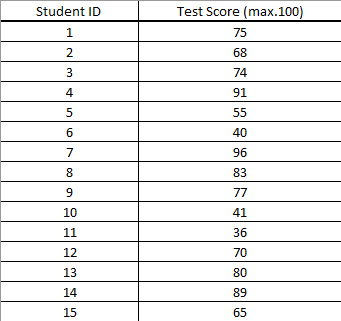

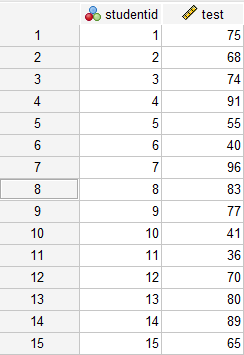
Before conducting a one-sample t-test, two criteria must be met. Firstly, the data should be interval or ratio, as opposed to ordinal or nominal, which would require a non-parametric procedure. Secondly, the sample data should approximate a normal distribution, which can be tested using SPSS Statistics. These considerations are crucial for methodological planning and statistical analysis.
For normal distribution (normality):
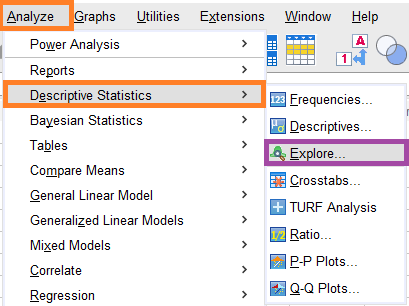
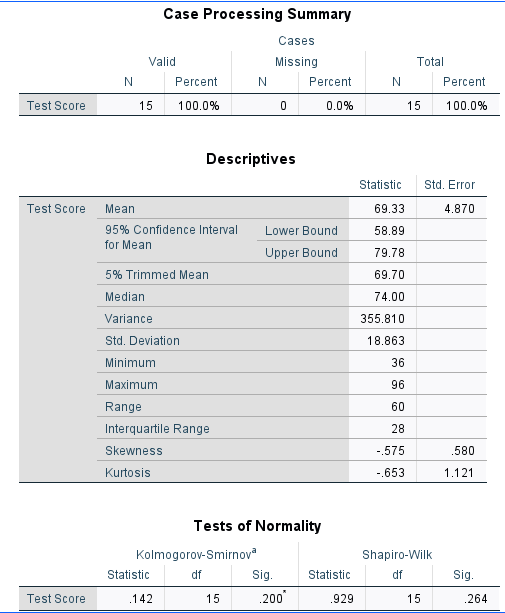
The Skewness and Kurtosis statistics describe the shape of the distribution of test scores. When both are zero, it indicates a “normal” distribution. By dividing these values by their respective standard errors, we can calculate z-scores. These z-scores help us determine the probability of a sample with extreme skewness or kurtosis originating from a population that follows a normal distribution, using the standard normal distribution as a reference.
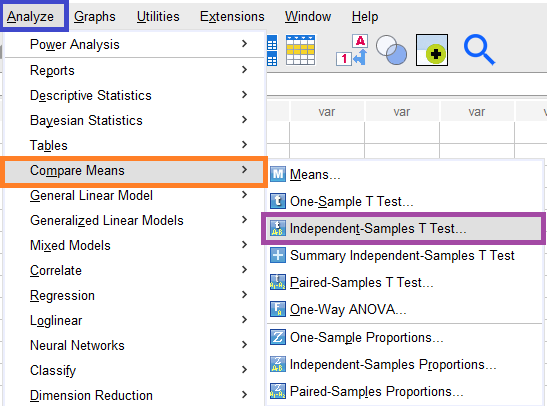

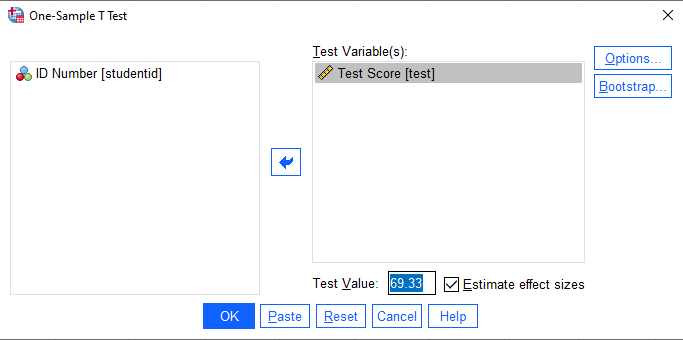
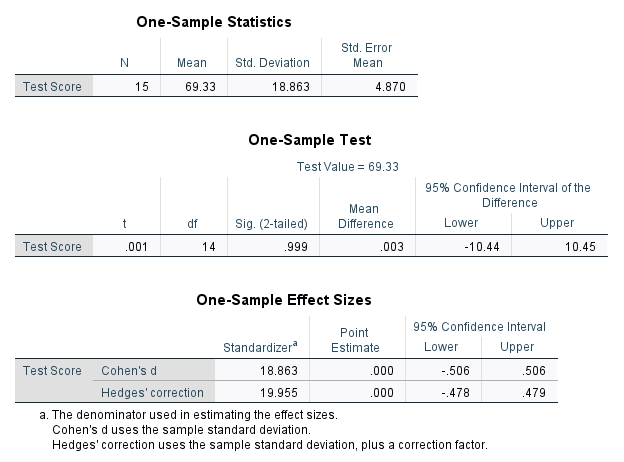
To help readers grasp the significance of a study’s results, it’s important to report the effect size. Cohen’s d is a helpful measure for indicating the magnitude of the difference between the sample mean and a reference value, like the population mean or a test value.
Independent Samples T-Test
To determine if there is a significant statistical contrast between the means of two separate samples.
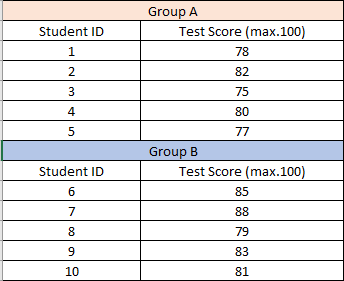

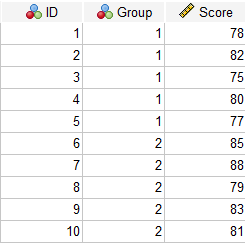
Before conducting an independent samples t test, certain assumptions need to be met. The first two assumptions relate to methodology and should be addressed during data collection:
1) The dependent variable (DV) should be interval or ratio data, and
2) Each participant should only participate once and not influence others.
3) Normality, where each group of scores should be approximately normally distributed, and
4) Homogeneity of Variance, meaning there should be similar variability in each set of scores. Normality can be assessed through various methods such as the Shapiro-Wilk test and visual inspection of histograms, while homogeneity of variance is tested as part of the t-test.
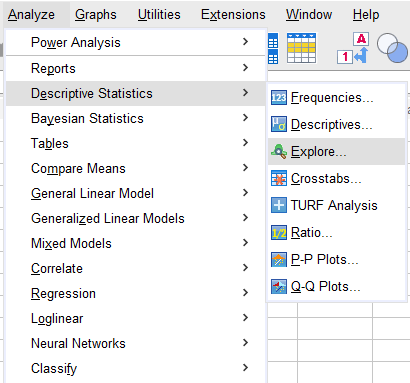